Added Information
Here you can find additional information about the processing we used for our analysis.
Different MBTI Types
Type | Personality Description | Most Grossing Character | Image |
---|---|---|---|
ESTP | Smart, energetic and very perceptive people, who truly enjoy living on the edge. | Lyle Wainfleet from Avatar | ![]() |
ESFP | Spontaneous, energetic and enthusiastic people - life is never boring around them. | Jack Dawson from Titanic | ![]() |
ENFP | Enthusiastic, creative and sociable free spirits, who can always find a reason to smile. | First Officer William McMaster Murdoch form Titanic | ![]() |
ENTP | Smart and curious thinkers who cannot resist an intellectual challenge. | Molly Brown from Titanic | ![]() |
ESTJ | Excellent Administrators, unsurpassed at managing things or people. | Colonel Quaritch from Avatar | ![]() |
ENFJ | Quiet and mystical, yet very inspiring and tireless idealists. | Neytiri from Avatar | ![]() |
ENTJ | Bold, imaginative and strong leader, always finding a way - or making one. | Voldemort from Harry Potter | ![]() |
ISTP | Bold and practical experimenters, masters of all kinds of tools. | Jake Sully from Avatar | ![]() |
ISFP | Flexible and charming artists, always ready to explore and experience something new. | Rose Dewitt Bukater from Titanic | ![]() |
INFP | Poetic, kind and altruistic people, always eager to help a good cause. | Luna Lovegood from Harry Potter | ![]() |
INTP | Innovative inventors with an unquenchable thirst for knowledge. | Grace Augustine from Avatar | ![]() |
ISTJ | Practical and fact-minded individuals, whos reliability cannot be doubted. | Tsu'tey from Avatar | ![]() |
INFJ | Quiet and mystical, yet very inspiring and tireless idealists. | Mo'at from Avatar | ![]() |
INTJ | Imaginative and strategic thinkers, with a plan for everything. | Oppenheimer from Oppenheimer | ![]() |
If you want to learn more about the the character types and the resources used on this page click here.
Genre Processing Technique
Our dataset comprises of more than 70000 movies, the majority of which are missing data in some form or another. Interestingly enough, it seems that most movies (roughly 3/4) were released after 1960. We first started by filtering out obvious outliers related to the release date of the dataset (everything released after 2012). We then decided to also remove revenue outliers, two movies whose grossing was above 2billions usd. One of them was avatar but the second is unknown (likely titanic). Since our analysis is mainly genre based, it is imperative that we first delete any movie missing genre data. It turns out that the number of different genres has been greatly increasing over the years. Overall there exists about 363 different genres (362 if we ignore the comdedy grammatical mistake). Drama, comedy, romance, action and thriller are obvious kings. Another genre that is dominantly present is black and white but that makes sense since first cameras could not capture colour. This filtration process is not limitted to removing movies with no genres. Indeed, we must also remove movies with aberrant numbers of genres, most notably a 17 genres movie. We therefore removed any movie with 10 or more genres and any movie without any for a total of roughly 2000 movies.
For the plot data, we first started by counting the number of sentences and the number of word tokens in each plot. The logic behind this step is that a plot containing too few sentences and words at the same time contains too little information to be relevant. By doing some statistics on the number of words per sentences, we first saw that the distribution of words per sentences is exponential. This also allowed us to find obvious outliers (2 words per sentence and below). After printing those outliers, we realized that some plots were not plots but rather were a list of the cast. Since this mistake repeated itself in non english movies, we decided to filter out all movies whose language is not english. Doing so had one major advantage which was that now the plots dataframe was half its size which would speed up the preprocessing steps. Interestingly, we only lost about 20 genres through this filtering process, which was statistically significant (proved by wilconxon test) and those 20 genres were mostly ethnic genres associated with different nationalities (ex: Tamil movies or Philippino movies). We still had some outliers (in terms of word per sentence) but at that point the first quartile was 17 word per sentence. We therefore filtered away any plot with less than 8 words per sentence (2 prepositions, 2 nouns, a verb, 2 adjectives and one adverb) .
Regarding characters, we described the statistics of height and age since most plots were unreadable. We discovered that some numbers are in feet for height which insinuate a 500m giant and a good fraction of the actors is aged 0 or less. We decided to set obviously aberrant values to nan. After investigating age vs height we also set the age of every actor aged 10 or below with a height above 1.5m to nan. We did not touch child actors nor actors afflicted with dwarfism.
Finally, we decided to investigate the male to female ratio over the years. Turns out that at the very beginning of cinema, this ratio was relatively smaller but it eventially started stabilizing around 3 (or 4). The average number of different ethnicity over the years has been steadily increasing.
LDA Technique
As promised in the main text here are all the different extracted topics from the investigated genres.
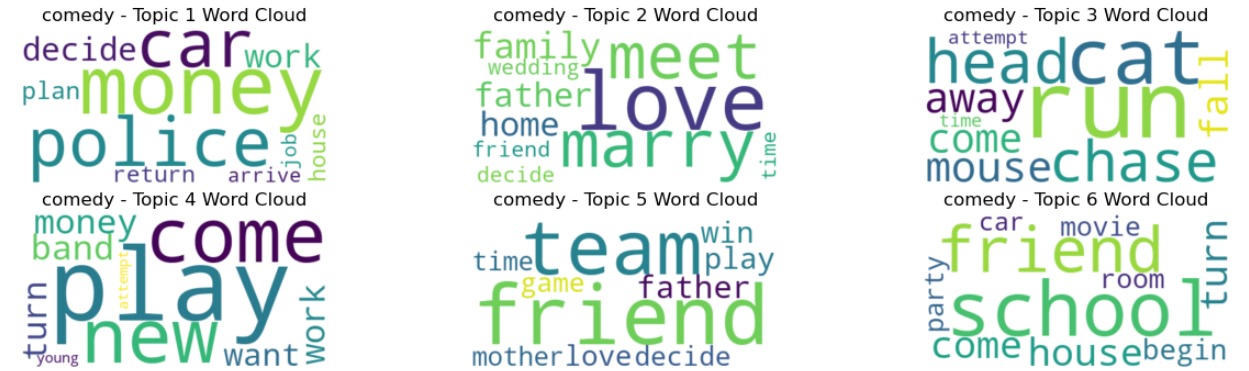
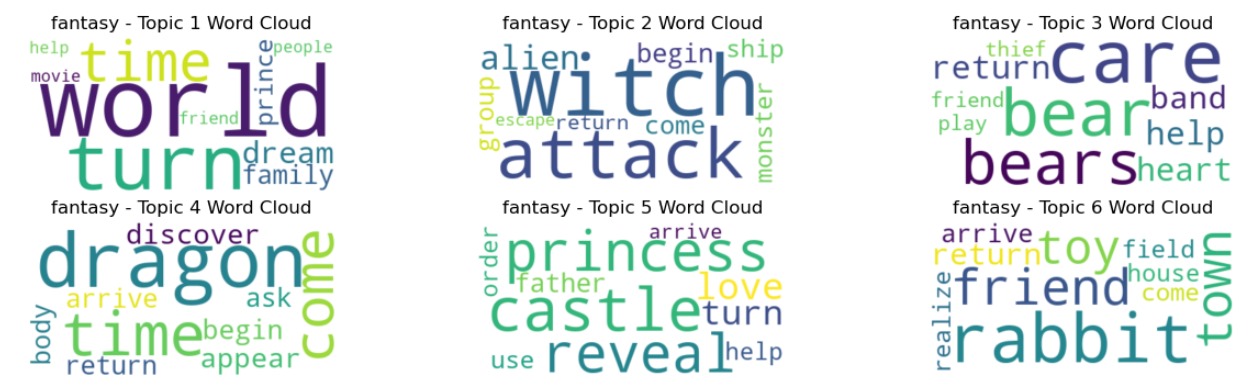
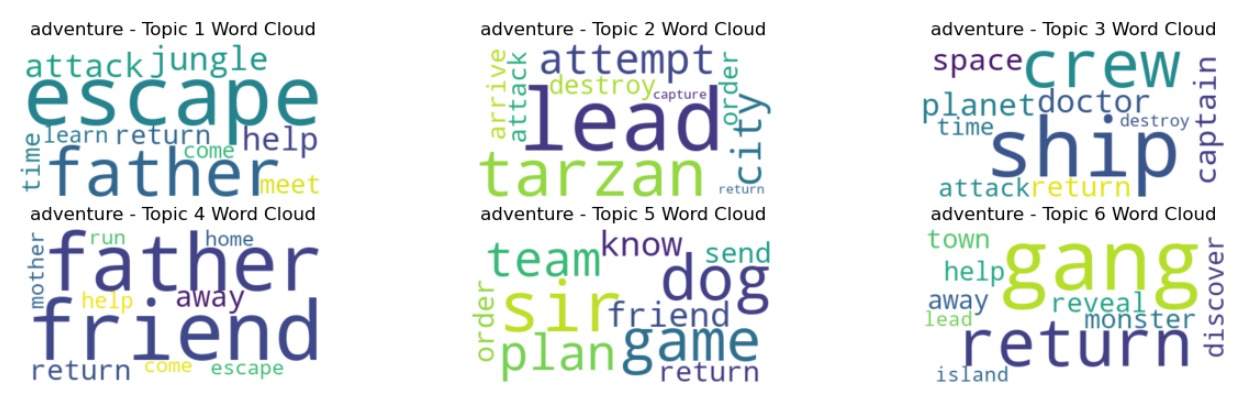
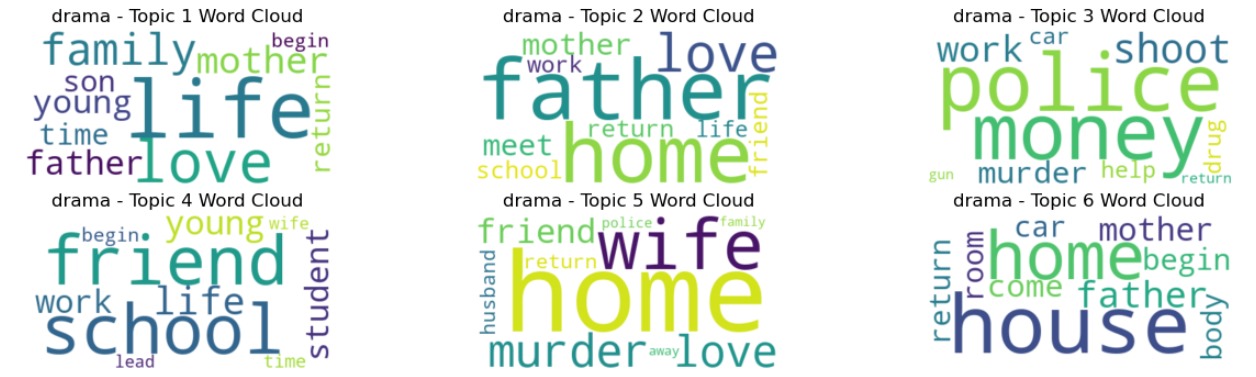
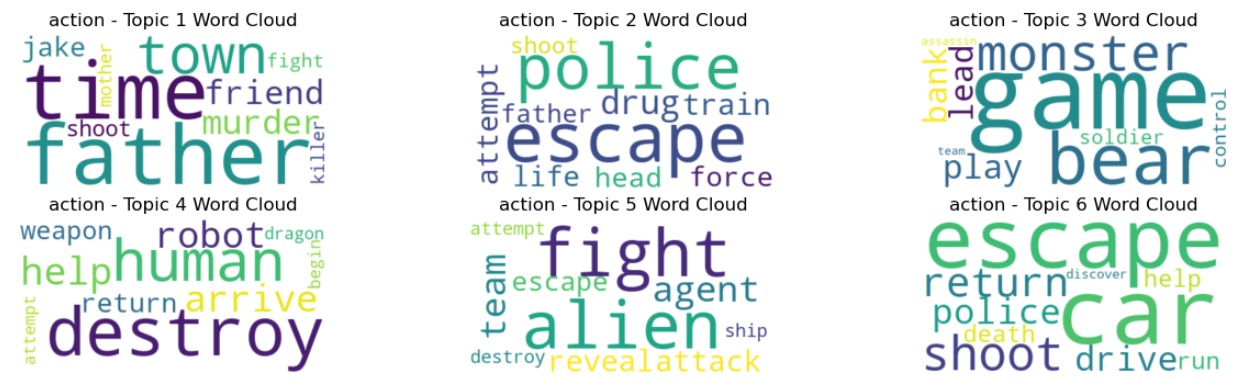
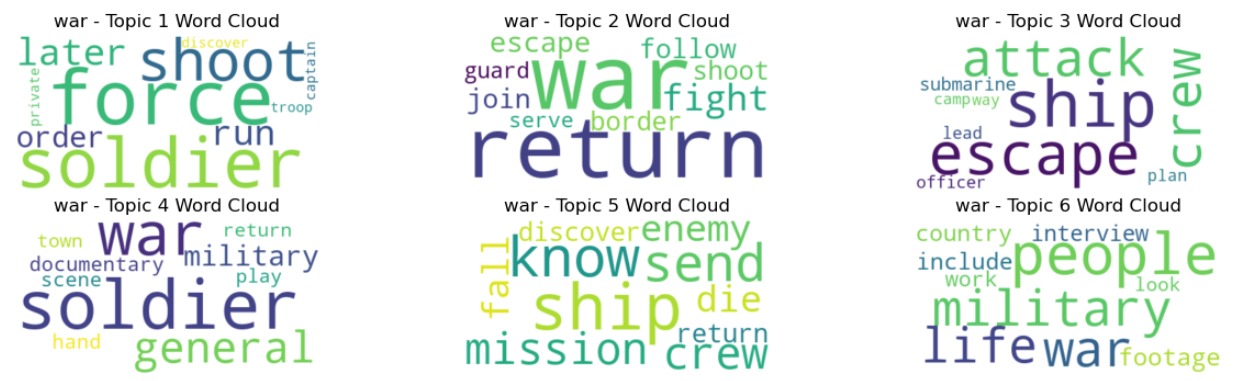